On September 21, 2021, Liu Lei's group from the Intelligent Medicine Institute, Fudan University,and Fei Shan's group from the Department of Radiology, Shanghai Public Health Clinical Centre published a research paper entitled "DeepSDM: Boundary-aware pneumothorax segmentation in chest X-ray images" in Neurocomputing. In this work,the researchersproposed a boundary-aware image segmentation algorithm that can effectively perform pneumothorax diagnosis based on chest X-ray images.
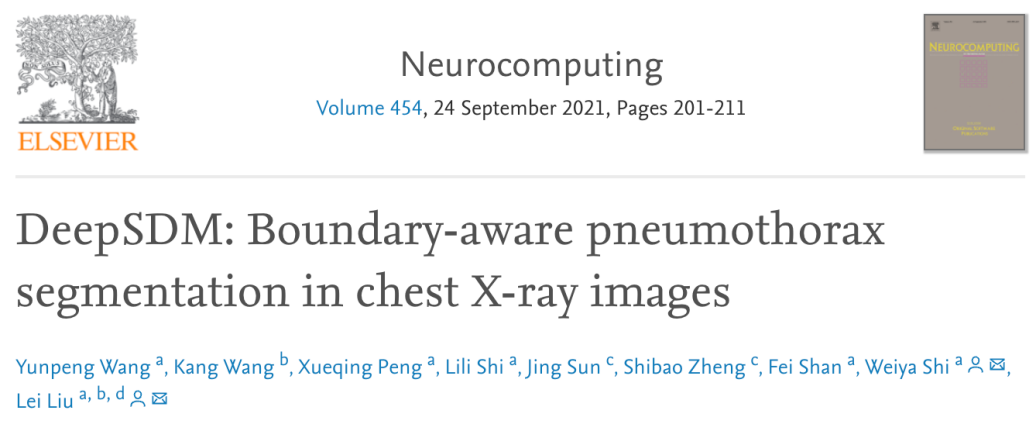
Fig1the first page of the paper
Pneumothorax is a common medical emergency defined as the abnormal collection of air in the pleural space between the lung and chest wall. Its typical symptoms include chest pain and dyspnea, leading to oxygen deficiency or even life-threatening in severe cases. Therefore, the accurate and rapid diagnosis of pneumothorax is of great significance in the emergency department. In clinical practice, radiologists usually evaluate pneumothorax on a chest X-ray. However, this process is highly dependent on the experience of the radiologist and can sometimes be very difficult. Moreover, not all imaging departments have doctors on duty all day long. Therefore, an efficient and automatic pneumothorax diagnosis algorithm would be useful in many clinical scenarios.
Existing automatic diagnosis algorithms for pneumothorax can be roughly divided into rough localization and accurate segmentation methods. Classification, detection, and weakly supervised object localization are three typical rough localization paradigms, They need image-level or box-level annotations, and only provide a rough diagnosis of pneumothorax. By contrast, accurate segmentation provides pixel-level predictions and offers more interpretability. Moreover, it enables the estimation of air collection size in the pleural space, which helps in drawing up the subsequent treatment plan. However, the problem of blurred boundaries of pneumothorax on chest X-rays is a common challenge faced by image segmentation methods. The boundary-blurring problem refers to the low contrast between pneumothorax and other body structures on chest X-rays, which requires even professional radiologists to adjust the window width and window position several times to distinguish the boundary of pneumothorax. For image segmentation algorithms, the blurred boundary problem can cause unsmooth segmentation resultsoreven loss of air regions in the area calculation.
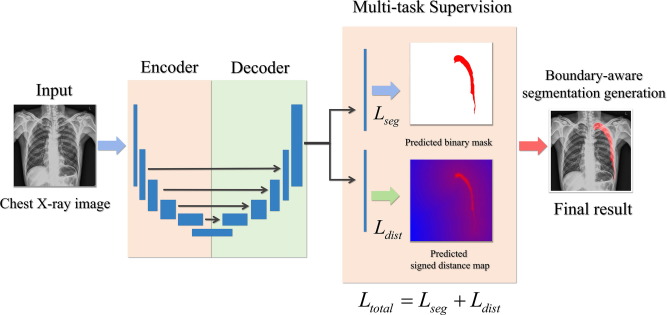
Fig 2 DeepSDM method
In this work,the researchersproposed a new learning framework, called DeepSDM, employing the rich information in the Signed Distance Map (SDM). The binary segmentation mask and SDM were learned in parallel via the multi-task strategy. The ablation study showed that this strategy effectively improved the segmentation performance compared with training on the binary mask alone. Furthermore, a boundary-based weighting scheme was adopted in the SDM regression task, forcing the model to pay more attention to pneumothorax and its contour. The experiments demonstrated the superiority of the boundary-based weighting scheme compared with a fixed equal weighting scheme. Besides, theresearchersalso experimented with several common multi-task learning structures and released a new multi-center pneumothorax segmentation dataset, called PTX-498. It included 498 pneumothorax chest X-ray images from three hospitals with pixel-level annotations. Extensive experiments were conducted on the Kaggle SIIM-ACR Pneumothorax Segmentation dataset (with 12,089 chest X-ray images) and the PTX-498 dataset. The DeepSDM showed better performance than the previous state-of-the-art in all evaluation metrics and generated more continuous and smoother segmentation results.
Yunpeng Wang, a Ph.D. student in Lei Liu's research group, was the first author of the paper, and Weiya Shi, Director of the Department of Radiology at the Shanghai Public Health Clinical Centre, also made important contributions to the work. The research was supported by the National Natural Science Foundation of China (#91846302), the National Key Research and Development Program of China (No. 2016YFC0901900), the Shanghai Municipal Commission of Health and Family Planning, China (No. 2018ZHYL0104) and the National Key Research and Development Program of China (No. 2016YFB0201702).
Original article link:https://doi.org/10.1016/j.neucom.2021.05.029